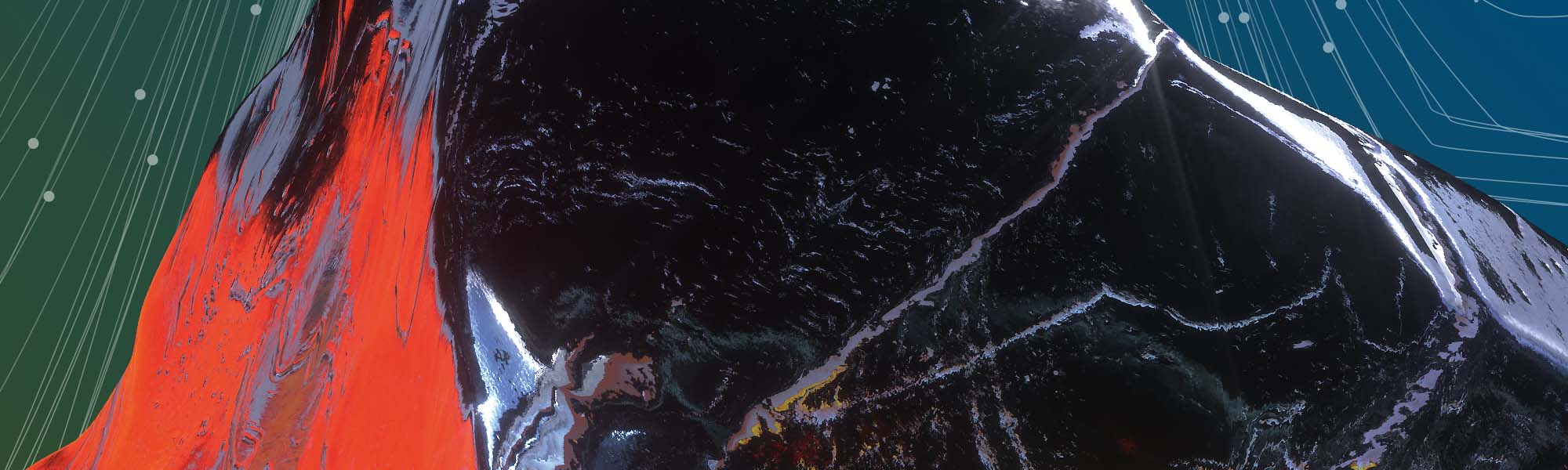
Columbia Road: What kind of engagement are you trying to generate at Zalando?
Saku: We want to inspire customers with meaningful fashion moments in our digital experience. It starts with the traditional click engagement as a sign of interest; a transition to another step like clicking through to a different catalogue page or product details. Beyond the clicks we try to understand what happens afterwards and include things like leaving feedback, following a brand, adopting a new trend or watching a video — so it’s quite broad from a customer behaviour perspective. Over time, we want to understand what kind of engagement will retain customers.
There’s a lot of content on Zalando’s platform. We’re building, maintaining and optimising a system which defines what content, for example, is shown on an individual customer’s home page when they land at Zalando. The content can be ads and articles from different teams at Zalando, from brands or some other third parties. What’s critical for us is to optimise the system so that the content is being shown in such a way that each piece of content provides as much engagement and revenue impact as possible — it’s all about personalisation and performance metrics.
CR: What are your KPIs in terms of engagement?
Saku: We have been learning a lot through this journey, and we’ve identified the key dimensions that contribute to our success in terms of content. The first two are how tailored customers perceive the experience to be and how big a share of the journey is tailored. The other two are relevance, which is to do with how well we’re able to pick the right content, and variety, which is how much content we have to choose from. Based on those, we had to figure out how to measure them and which aspects we can directly influence and which we can’t.
We identified a hierarchy of KPIs. We drive the group level north star metric — customer lifetime value - with engagement and retention. So the direct top level KPI for us is the number of engaged customers over a certain period of time. Then we have different outputs that matter for engagement, such as the share of paid advertising content, share of content that matched our customers' fashion preferences and what is the total predicted page value. These are based on content’s expected contribution to engagement, advertising revenue and transactions. To match the outputs we have input KPIs that describe the breadth of available content offering and how much we understand about the customer. What matters here is that we have a solid understanding of what we and Zalando as whole can control vs what is external variance.
CR: The Zalando platform is really big, with multiple propositions, categories and country sites, so managing the complexity of the data sounds challenging. What processes do you have in place to address this?
Saku: There’s this phrase that “if you build it, then you run it”. For the systems we now have that should perhaps be “if you build it, then you understand it”. We need to understand the input and output KPIs that are part of the request and what we can build and aggregate on. Privacy is a really big chunk of managing this. The experience of trust and respect for privacy is one of our six customer promises. We do not just want to be compliant but a leader in this area. That has been a heavy investment area in the past few years — making sure that the recording and processing activities are stored and only accessible by people who really need it. It takes a lot of work to keep our data management compliant with regulations.
CR: In short, how do you make sure that individual pieces of data are accessible and being utilised in a responsible way from different parts of the system and organisation?
Saku: We have efficient ways to see what data is available. We have thousands of data streams relating to tracking and different services, and you can search what’s available and find out whether the data contains personal identifiers. All the data streams also have a schema description. The streams and data are classified into different confidentiality domains and depending on the domain the access is restricted. If we share anything — even between different teams — it has to be properly aggregated and anonymised; the only way to have access to the confidential data sets is through a strict process.
CR: Getting to that stage is something that many companies can only dream about! Is it a complex challenge to make sure that the data is utilised in a compliant way and that its quality is trustworthy, and also that people understand what data they can use and how?
Saku: We’ve been working really hard to ensure that we only track the essentials and that there’s no bloat where we’re tracking things that we don’t need. Later, we can explore the dimensions in the metadata that are part of the content so we can correlate it later rather than including it in the tracking mechanisms. This way we can add more dimensions in the tracking data much faster than would be possible with traditional methods where everything is put in the tracking information. That’s pretty handy, as there are global unique correlation keys that we can use across customers and tracking errands. This also helps to keep us compliant as we can drop all the tracking data that we can’t use.
CR: What experts do you have in the team?
Saku: We have around 10 data scientists, around 20 engineers, five product managers and also focused analytics and design teams that work with our team, who are part of the more than 2,500 employees working in tech at Zalando. In terms of profiles, some of the software engineers are more focused on the frontend topics, while some are more focused on data engineering. We’ve split these topics around the input KPIs that we have. There are people who are focused on understanding the economics — the inputs and outcomes. Then we have teams focused on tailoring the experience and connecting with the customer. We’re also responsible for the understanding of the content.
We are additionally building a content platform that provides a really quick way to experiment with new content without worrying about operational issues or content delivery. This provides a way to track content so people don’t have to worry about tracking, but they get the correlation keys and can then use their own metadata to attach to it later. We’ve ramped it up from one to 15 content formats and thousands of new entries every week in six months, so building it was a good exercise.
CR: Has the challenge of getting such a sophisticated content platform up and running been more about tackling technical challenges or about collaboration between teams?
Saku: We believe that the more content we have from different types of contributors, the better we can make the experience. We considered our role and came to the conclusion that it’s more about content acquisition than content strategy and content production, but we need to make it as easy as possible for teams to build their content products and for external partners to contribute content, so that it’s not a huge investment for them to understand if the content works or not. The thing we had to tackle there was that before this setup, everything would typically have its own data structure. So whenever there was a new piece of content we’d need to do some kind of transformation to understand what’s included.
Providing a good publishing and delivery pipeline is quite challenging on this scale, especially with the latency, resilience and localisation expectations, so we actually solved this pain for other teams. We’ve been able to get to a point where everyone’s using one unified data structure in terms of how the metadata is labelled and how long it’s available. This has been done to have solid data contracts with all the content producers, that describe the content types while giving them the flexibility to use whatever they want to present their content in the way they want to.
Saku: The final push was not that difficult but we spent a year and a half to understand what our organisation's role should be, and what are the problems we need to solve for others. It took a while for us to feel confident that we had a good enough understanding of why and how to do this in a way that would be worth the investment and benefit the other teams so much that they’d want to get involved.
CR: It must have taken quite an effort to combine all the different points of view and aspirations across the organisation?
CR: Coming back to the team, you said that there are experts like data scientists, data engineers and designers. Do they all share the same objective of engagement, or are they more like virtual teams coming from different points of view and supporting your team’s key KPIs?
Saku: We haven’t set them up in a way that all the data scientists sit in one team. The people who build the endpoints and services needed to access the predictions sit together with the people building the prediction models. Typically in a team there are a couple of engineers focused on how to build the services and make them resilient, then the data scientists work together with them figuring out how to build the system in a way that lets them experiment with different models. They work together to get all the data in one place so we can join and analyse it for decision making and to generate an understanding of performance.
CR: Typically the challenge for many companies seems to be that the data engineers are deep in the IT organisation and there’s an analytics team and frontend builders in other teams, and coordinating these teams is really slow. It seems like you have everything closer together so you can get something like AB testing done in the frontend when you need it?
Saku: Yes, that’s how the whole company is set up. You need to understand the customer and the problem that you’re going to solve for them, and then the principle is that you invest in enough people and build the right kind of team to solve the problem rather than a setup where you have to compete with some distant resources to do every small thing. This is critical for our success because for the products we’re building that are based on data and machine learning, it’s difficult to figure out when we will get the outcome, so there might be quite a few iterations. If we had to request one analysis after another until we get what we need, it would slow us down quite a bit and we wouldn’t be building the kind of domain understanding that we want people to have.
CR: What have you learned that you wish you’d known from the start of this process?
Saku: If I think about how much it’s changed from optimising tens of pieces of content each visit to thousands, and simultaneously constantly improving the customer experience so we can measure it based on engagement and enabling significant advertising at the same time, we’ve actually built something quite compelling over the years. We already understood the dimensions that affect our success early on, but if I were to start again now I’d put more emphasis on rigorously iterating and on understanding how to measure those dimensions. Also, I’ve learned to keep it simple in terms of being clear on the ultimate objective the product should be optimised for.
Typically, for any product that’s creating the customer experience on ecommerce platforms, different stakeholders will have different opinions on what it should be optimised for, whether that be immediate conversion, driving profit contribution or solving inventory problems. Where we’re lucky is that our board understands the complexity of these issues and we have a clear understanding of where we’re going.
CR: I liked your point about how it’s not only about understanding what works, it’s also on a meta level, understanding what affects how you understand what works.
Saku: Yes, we could consider our KPIs as really well defined, but understanding the causalities between inputs, outputs and outcomes will help us understand what to focus on. At least for more mature setups that want to drive long-term success in ecommerce, I can understand the desire to work with objectives and key results (OKRs), but if you want to learn more about what makes an impact, first you need a better understanding of the long-term drivers for achieving your goals, and then you can approach it with the OKRs and see which parts you want to improve.
CR: On a high level, what were the key obstacles you had to overcome when you started running AB tests?
Saku: To tailor the content experience with machine learning algorithms, we ran the first AB tests around four years ago. The initial obstacle was that people were afraid of losing control. It got easier after very positive results, but at each step we had to communicate that we were first running an experiment, and that’s the key part. There are multiple stakeholders who want to display content or get their share of customer attention, and we have to explain the impact of intervening with the system. We’re constantly measuring the ideal system, where no one can intervene at all with the algorithm and it only makes decisions for the best customer experience, then we’re comparing that against the scenario where we enable marketing investments and advertising. We work with the stakeholders to educate them on what works and which parameters they can tune to create maximum impact from customer attention without hurting the overall customer experience.
CR: From a system perspective, I can imagine that there are hundreds of data sources and data lakes and machine learning functionalities. How were you able to get concrete results while maintaining coherence?
Saku: We’ve had our fair share of challenges on that front in the past. You need to create different kinds of monitoring — first, to find whether we got the data and if the model training went through, and if we are getting any errors in actually scoring or ranking the content. Then you need to constantly improve so you can start detecting deviations in the calibration of the predictions — so, we monitor things that explain not just if things are working technically, but if they’re producing the results we expected in a business and logical sense. If we start with a specific app version on a specific platform, do we start seeing less tracking events in some dimensions and can we drill down to find the anomalies if we see anything changing? I think we’re doing pretty well there, but when you think about the amount of data, systems and events, there are also some moments when things fall into the grey areas between teams or departments. Whenever we discover another one of those we can figure out a solution.
CR: Let’s talk quickly about customer lifetime value (CLV) — it must be complex in your setting?
Saku: Yes. We tried to optimise the content based on post-click revenue within the same system, and you’d think that this is a relatively short part of the customer journey. We gave it quite a few attempts, but in the end predicting the immediate engagement for the content turned out to be more impactful than focusing on the conversions on post-click revenue. The customer journey is already so noisy over this short span of time that it’s actually rather difficult to optimise it like that.
Now, our approach is more to understand the long-term drivers of customer value, so we try to include things like how big a share of their wardrobe has come from Zalando or what kinds of beauty brands they follow in the evaluation. In this way, instead of trying to optimise for immediate revenue or transactions, we try to optimise for events that are even further away but that have solid causal economic research behind them. Then we use that along with our prediction of customer engagement to come up with an approach that lets us optimise the lifetime value.
This work is in the early stages, but it’s showing some signs of success already. We’re trying to build models where we can understand long-term CLV, say 90 days, and then we’re working towards learning the parameters that affect the 90-day CLV. First we need the parameters and then we need to run the system for quite a long time — so we’re on that path but it will be a long journey.
SAKU LAITINEN is tasked with driving engagement and inspiration by matching content to customers along their journey on Zalando, a leading European online platform for fashion and lifestyle. He and his team are also responsible for enabling the monetisation of engagement through advertising within the digital experience.