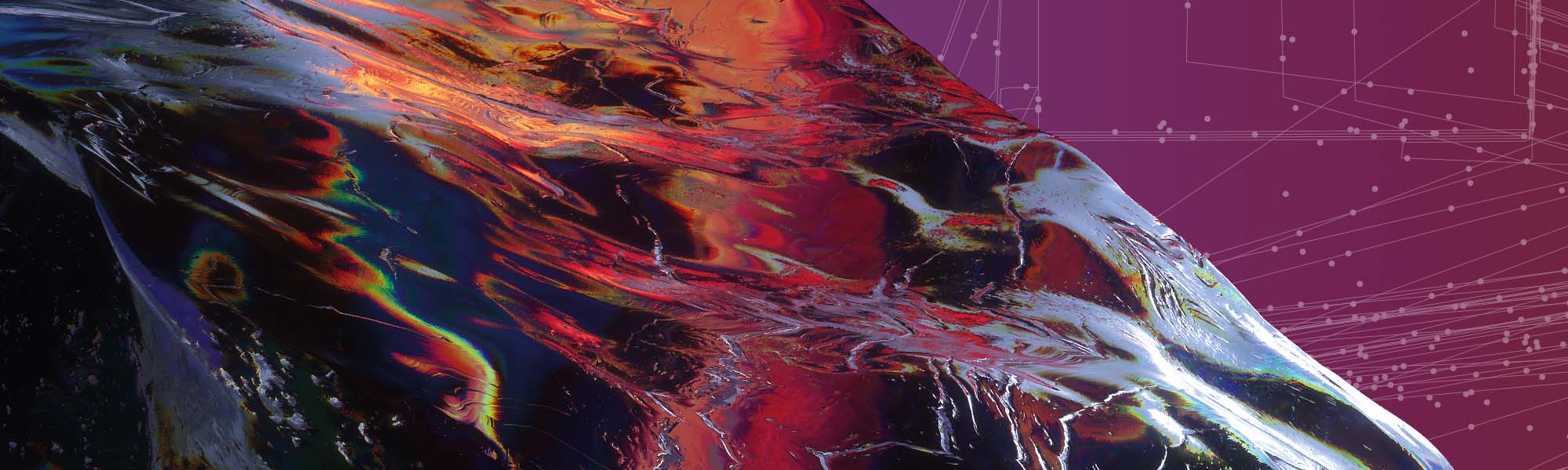
Columbia Road: What are the challenges that your clients have recently been tackling around more advanced data analytics?
Supermetrics: In our experience, there are three main challenges facing the marketing space at present. The first of these is a skills shortage, in terms of people who have a combination of data science skills and business knowledge and can use this to generate insights. Then we have the challenge of data stability, which relates to things like transformation, response time, data structure and gathering data from multiple sources, along with the ever-increasing complexity and volume of data in marketing and ecommerce. The final challenge is marketing measurement where attribution has been a challenge with the growing focus on privacy and an increasing lack of trust.
CR: Can you tell us more about these challenges?
Supermetrics: Sure!
Skills shortage
So the first challenge is finding people with both the technical skills in terms of data science and the business understanding required to draw insights and make decisions based on the data. There is a real shortage of people who can do both, leading to a mismatch between the data science team’s output and business decision makers’ expectations, as well as a lack of sufficiently accurate analytics that actually fit the business need. There are a few approaches that companies might use to address this.
One method is by carrying out internal training, although this takes time and still doesn’t always cover the gap. Another trend is automated machine learning, as this frees up data scientists to focus on solving business challenges. We’re also starting to see new business intelligence solutions, which are often self-serve and contextual — you can ask a question and get the answer. Some of these new trends will address the skills shortage and can also help to democratise data analytics.
Data stability
That leads us onto our second challenge — with the way the landscape is developing, there’s a huge, and growing, volume of data that quickly becomes an unmanageable mess. Data engineering capabilities are critical for making the data sensible and accessible and keeping it that way. However, instead of investing in these capabilities, many companies focus primarily on getting data scientists in, which leads to them getting frustrated with the slow pace when it comes to unearthing valuable insights that will see their work generating dollar value in the bottom line.
Yes, there are lots of applications for data scientists that can impact the top line, but all of those depend on having a stable data infrastructure, so hiring them isn’t necessarily a solution in its own right. First, you need to have data engineering to provide them with a baseline of high-quality, accessible data.
Attribution measurement
Because of privacy challenges*, third-party data hasn’t been reliable for some time now. That has led, once again, to more focus being placed on measuring impact through traditional marketing mix modelling (MMM), which helps not only from the privacy perspective but also with brand-related activities. The increased focus on privacy is changing how marketing teams work and think about data — in the long term this will force them to rethink how they can add value for the customer while working in a more ethical way.
The other thing is that attribution is not going to work in this new world of privacy, which paves the way for using lift testing to decide where to allocate the marketing budget. A lift test is a bit like an AB test that measures the incremental impact of marketing dollars when they are allocated to certain activities. They are not that straightforward to do, however — they can take a long time and a partnership with an ad platform is required, as well as people with the right skills. This means that smaller companies will miss out on the benefit of lift testing, which opens up an opportunity for someone to offer a platform that can automate it. There are already some tools and methods available that can bridge the skills gap and help to democratise data and make it available, like Power BI and Tableau.
CR: You mentioned marketing mix modelling — can you explain more about what that is?
Supermetrics: This isn’t really a new topic in the sense that MMM has been there since TV advertising began, long before digital ads came in. It’s a statistical approach to understanding the impact of your marketing dollars across different media. With increasing third-party cookies, tracking and so on, the importance of MMM diminished but it’s making a comeback because of the limitations of attribution. With MMM, you can track each touchpoint where your marketing dollars are allocated, looking at all the different inputs including spend and other factors such as the weather. Tracking those data trends then allows you to understand the long-term impact of spend on particular activities over a given period of time. MMM has its drawbacks, for example it doesn’t help on a day-to-day level, but it’s coming back into prominence because of the privacy-related restrictions around tracking.
CR: What are the current opportunities related to data?
Supermetrics: With so many metrics and so much data, one thing we’re currently working on is establishing what metrics are important. Understanding which data should be used to make decisions is a big thing, and to accomplish that you need the right skillset – as the data grows, having the business knowledge to make use of it becomes increasingly important. In the end it’s all about supporting growth and sales. The real opportunity is to find ways to collect data, make sense of it and structure it in a way that’s easy to analyse in order to gather insights that can better inform marketing and the other teams who rely on it. If we can close the ecommerce reporting loop – so breaking down the data from the perspectives of acquisition, how people behave in your online store and how we turn that into sales — then we can model the customer journey, looking at our KPIs at different stages and finding opportunities to grow. On a more advanced level, MMM gives you a better idea of how your marketing is really performing, as opposed to things like multi-touch attribution where you never get a full picture of what’s happening.
CR: How should companies go about building a data science team?
Supermetrics: This really depends on the company’s maturity level in terms of analytics — the crawl, walk, run philosophy comes into play here. For instance, a lot of leaders hear about advanced topics when they go to conferences or read articles, but the company may not be mature enough to approach those things yet. Another consideration is organisational structure — do you go with a centralised team that caters for all your analytics needs, a completely decentralised team with analysts, product and marketing teams working separately, or a hybrid of these two approaches? You need to know which structure will work best right from the early stages of this journey.
In general, in the initial phase the focus should be more on engineering, making sure the company has the right data and that it is stable and available. Then you can build on top of that with business analytics and business intelligence. Once you’ve started generating value from your analytics and business intelligence teams, you can add in predictive algorithms, personalised advertisements or even location intelligence, and then you can get to data science after that. The workload for this is huge, so before investing in building a data science team there should be a clear roadmap in place for identifying what you need and then building a team to answer these needs, rather than building a team and assuming something will come out of that.
CR: Can you share any concrete examples of a company using data to make decisions?
Supermetrics: One example was at my previous company, which sends out lots of customer emails. Analysing through different touchpoints allowed us to better understand our customers’ behaviour, building this understanding into a more individualised approach and changing how many emails we sent them per week. By providing the right messages at the right times we could reduce the number of emails we were sending out overall without losing impact.
CR: What are the main challenges around managing multiple data sources for marketing and ecommerce, and how do you help your customers with these at Supermetrics?
Supermetrics: The biggest challenges are the cost of maintenance and the speed of execution — the data isn’t automatically available quickly enough from different ad platforms that the marketing team is trying to explore, and that’s where Supermetrics comes in. We’re market leaders in that sense. In terms of maintenance, every time platforms like Google, Facebook or LinkedIn change something, our customers don’t have to worry because we’ll take care of it — from that perspective, Supermetrics is an all-encompassing platform that makes sure data continues to flow.
One of the ways we segment our audience is between data managers who build and maintain the infrastructure and data users – including marketing teams — who come in and work with the data. Supermetrics can support both of these segments — this then brings us to the build versus buy question. If you’re building your data infrastructure then the onus is on you to maintain it and fix things, which can be full-on at busy times such as Black Friday. Supermetrics can take care of that aspect for our data manager customers, which gives them peace of mind. And as for the data users, they know that they’ll have that data available, which is useful for them.
* Stricter privacy legislation, such as the EU’s GDPR, cookie consent requirements, California’s CCPA and browsers blocking third-party cookies. Third-party cookies have enabled user tracking, for instance for Facebook, other marketing platforms and web analytics software.
SUPERMETRICS streamlines the delivery of data from sales and marketing platforms into the tools and destinations that marketers — and the analysts and engineers who work with them — use to make better decisions, such as spreadsheets, data visualisation tools and data warehouses. Demand Gen Director Edward Ford, who joined the company’s marketing department in its early days, and Vishnu Sahoo, who in his role of Marketing Analytics Director mainly focuses on marketing analytics and group marketing, talk about the challenges they face, as well as opportunities and solutions in the data analytics space.